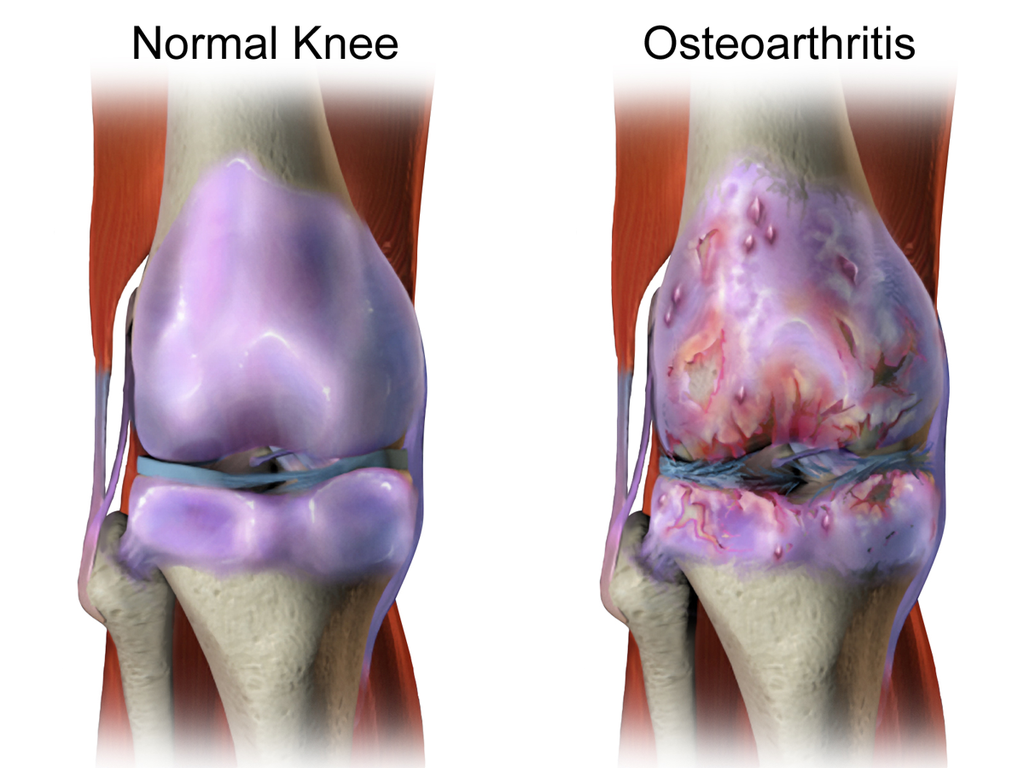
New algorithm predicts rheumatoid arthritis
Based on genetic data, a new diagnostic algorithm can differentiate between five different rheumatic diseases.
The team of Dutch and US researchers writes in Science Translational Medicine that they have developed a new diagnostic tool that can ease diagnosis of rheumatic diseases. The algorithm combines preexisting genetic data of a patient with his genetic risk scores to home into the most probable diagnosis among five different rheumatic diseases. These slowly progressing inflammatory diseases often exhibit similar symptoms early on, complicating a clear diagnosis.
The algorithm even demonstrated greater diagnostic accuracy than a rheumatologists predictions at a patients first visit, and showed that 35% of patients included in the analysis were in fact misdiagnosed by their rheumatologist at these early appointments. By potentially leading to quicker, more accurate diagnoses, the application of genetic risk scores in the clinic could help patients start therapies sooner, avoiding further debilitation or late-stage treatment with harsh immunosuppressants.
Genetic risk scores are not effective in predicting rheumatoid disease prevalence at the population level because of the rare occurrence of this disease type. By contrast, Rachel Knevel and colleagues demonstrate that genotype data can aid individual diagnoses in the clinic, where patients who present with symptoms of inflammatory arthritis may have a higher likelihood of being affected by rheumatic disease.
The researchers used their algorithm, called G-PROB, to discriminate among gout, lupus, rheumatoid arthritis, psoriatic arthritis, and spondyloarthropathy in roughly 1,700 patients with preexisting genetic and clinical data who had been diagnosed with one type of rheumatic disease and/or had presented early inflammatory arthritis in their first visit to the outpatient clinic.
The G-PROB platform could rule out at least one disease in all patients, identified a likely diagnosis in 45% of the patients, and caught incorrect clinical diagnoses in 35% of cases. Moreover, adding genetic risk score calculations to clinical data improved G-PROBs diagnostic accuracy to 51%, compared to 39% resulting from interpretation of clinical data alone. To further refine the algorithms precision, clinicians could incorporate non-genetic information made available at a first visit, such as family history and age.