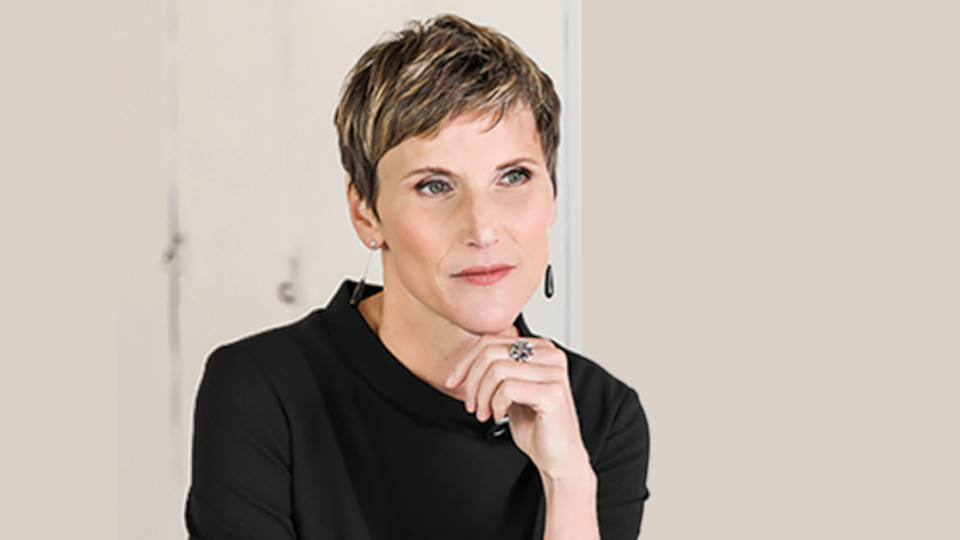
AI – tool or trouble?
Depending on who you talk to, artificial intelligence (AI) is either a saviour or an enslaver. The concerns range from loss of jobs through to robots running society. However, in the life sciences, the use of AI and machine learning (ML) have created some excitement over their potential applications.
While AI has been steadily evolving in the IT community for decades, for the general public it seems to have only recently made the leap from science fiction to reality. Authorities have identified the need for regulation of AI, while technology-savvy entrepreneurs have already created applications for both highly-skilled personnel and the average consumer, the most prominent example being ChatGPT.
This has led to an ongoing critical review of AI to investigate its uses in and effects on industry and society, such as cost savings, innovation and employment. New applications of AI are also steadily being developed, and data-intensive disciplines in the natural sciences, such as biology or medicine, can particularly benefit from the new possibilities, which enable users to process more data than ever before.
To demonstrate the impact of AI, Mount Sinai Hospital in the US is among a group of leading hospitals pouring hundreds of millions of dollars into AI software. They are buoyed by a growing body of scientific literature – such as a recent study finding that AI readings of mammograms detected 20% more cases of breast cancer than radiologists – along with the conviction that AI is a valuable component of the future of medicine.
As most scientists are neither programmers nor informaticians, a new consulting industry is budding. Supporting the evolution and application of AI in science, and providing the interdisciplinary expertise required to translate the story of any research undertaking into code, they become a gateway for accessing AI, supplementing a difficult-to-find expertise.
Applications in biotech
In the evolution of medicine, technology has always played a part. In the 1990s and early 2000s, AI algorithms began deciphering complex patterns in X-rays, CT scans and MRI images to spot abnormalities. Likewise, companies incorporated algorithms that scanned masses of patient data to spot trends when developing tailored treatments. AI has generally been welcomed by life science companies for its ability to work with massive amounts of data, and to generate data-driven results such as new drug targets.
Several AI-native drug discovery companies have progressed AI-based molecules into clinical trials, reporting greatly accelerated timelines and reduced costs, and raising high expectations in the R&D community. This performance has been discussed in a recent Nature Reviews article, where the disclosed discovery programmes and preclinical assets of the top 20 of these companies were compared with the top 20 of Big Pharma. Astoundingly, these young AI-native companies already have a combined pipeline with close to 50% of the number of assets that the top 20 of big pharma are running.
AI behind the curtain
Being involved in bringing many projects and innovations from concept to user, the BioLizard team has experienced and worked with a wide range of requests and ideas for the application of AI across the biotech and pharma space.
To give an example of how AI is implemented from concept to product, one recent project for a client was an end-to-end, data-driven solution for predicting clinical outcomes in transplantation patients. The main focus was on using RNA sequencing (RNASeq) data to predict different types of organ transplant rejection in patients. The task was to expand and improve the efficiency of the approach by providing an end-to-end workflow, from input of raw RNASeq data through to the prediction of transplantation rejection.
The data analytics & AI team first set out to create predictive models for both acute and long-term organ rejection using a dataset of 60,000 genes from 150 patients. However, the complexity of the data, compounded by the biological variability among different ethnicities, genders, and ages of the patients, at first made it difficult to derive meaningful biological insights.
Consequently, the team narrowed things down to a more manageable set of genes that were found to have the highest predictive value, and then worked with the client to choose a subset of clinical features that would also be included in the predictive models. This way, the resulting models would take into account not only information related to gene expression, but also other potentially predictive variables, such as the presence of patient comorbidities.
BioLizard improved and tested the models over time, and the final result presented to the client was a highly standardised and data-driven product that is now being validated for use in a clinical setting to predict patient outcomes. This case is a great example of how human input is still necessary to make the most out of AI: by selecting the best set of predictive genes and a complementary subset of clinical information, a client was being enabled to apply AI to its highest potential.
What are the limits of AI?
Regulation is a huge consideration in the development and use of AI. The FDA, in a recent discussion paper, acknowledged current and potential use of AI in the field, and agreed that AI/ML has the potential to accelerate drug development and make clinical trials safer and more efficient.
However, the importance of assessing if AI/ML introduces risks is also noted. For example, AI/ML algorithms could amplify errors and pre-existing biases present in underlying data sources and thereby, when the findings are extrapolated outside of the testing environment, raise issues related to generalisability and ethical considerations. These concerns have resulted in the development of standards for AI to address areas such as explainability, reliability, privacy, safety, security, and bias mitigation.
The European Commission also promotes the use of AI, while emphasising the need for data protection. The current draft framework of the EU Artificial Intelligence Act is still evolving, which is why the European AI Alliance has been created to provide a forum for open policy dialogue. Some of the concerns expressed by healthcare AI stakeholders have already been addressed, but one key issue remains. As it stands, provisions for governance of data appear to exclude most real-world data as a source of evidence. This would greatly diminish the applicability of AI in healthcare, as most data from the laboratory or clinical studies could then not be used to train AIs to develop predictive capability. At the time of publication, the regulator is still open for discussion to reach a pragmatic solution.
Another real obstacle to the application of AI can be misconceptions. AI is not magic, and cannot provide reliable results if not fed with the right data and human insights, and if the output is not interpreted correctly. As with all analysis methods, it is important to consider where the data comes from. If an experiment was conducted in a way that is not applicable to the question at hand, AI won’t be able to draw reliable conclusions. On the other hand, applying the vast amounts of public data resources available can be a path forward, when using an applicable and relevant, not necessarily perfect, data set as basis. Identifying the right input data for the question and ensuring careful interpretation of the output information – both of which may be challenging as this requires both biological understanding and expert knowledge in data science – is of crucial importance, and part of the expertise BioLizard is providing.
AI – Value for money
Another misconception that can easily be overcome is related to costs. Many consider AI to be expensive, while not considering that just like in the wet lab, it is possible to start with small proof-of-concept studies to see how and where AI will add value to a company. For this reason, BioLizard assists clients by examining their unique situations and pinpointing key areas that could be streamlined using AI/ML. While not always applicable, often opportunities for improvement arise, and BioLizard can help clients decide where AI can add the most value.
There can be little doubt that AI is here to stay and, as it evolves, it has the potential to positively transform the life sciences sector. However, regulation and risk mitigation are clearly important, and the industry needs to be a part of that ongoing discussion.
As with any new technology, utilising AI and ML appropriately and wisely is critical to successful implementation. This is why it is important to know who to turn to in order to navigate this new and exciting path towards the ultimate goal – a more efficient life sciences industry that provides new and better solutions for patients.
This article was originally published in European Biotechnology Magazine Autumn Edition 2023.