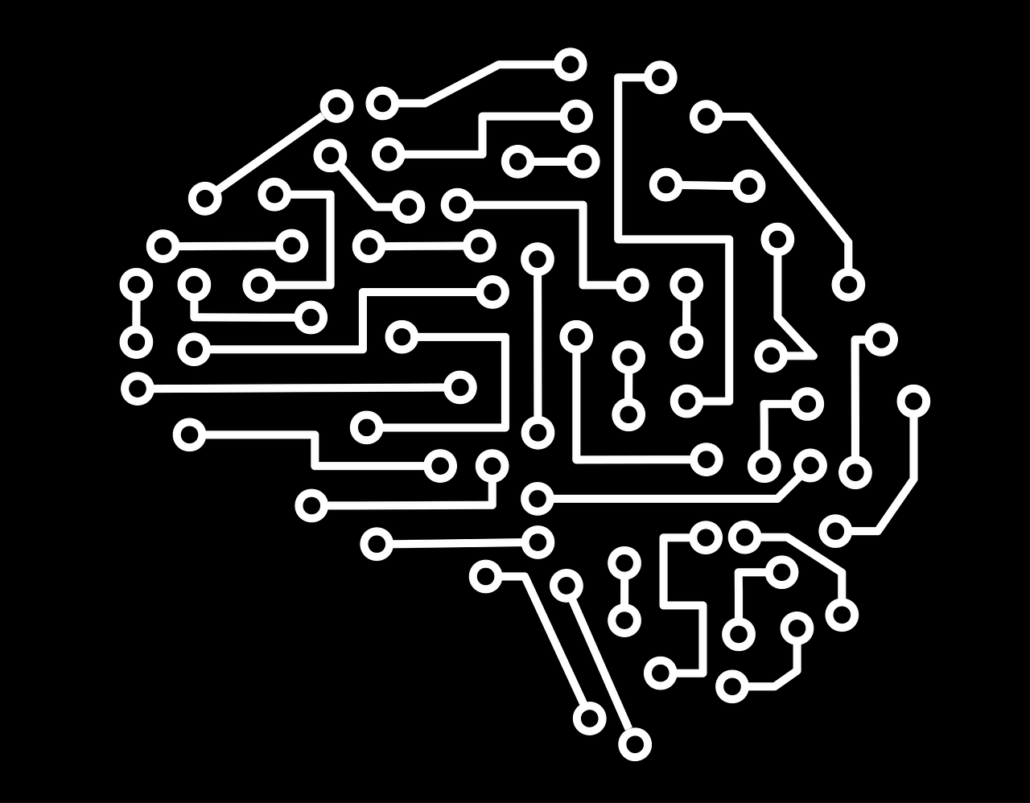
Researchers present AI-guided protein design tool
Danish AI expert Francesco Pesce and collegues have expanded the toolbox for computational protein design. Their new algorithm will facilitate the design of proteins whose functions exploit the many properties afforded by protein disorder.
A new machine learning model can predict the structure of intrinsically disordered proteins (IDPs) – a subset of erratically shaped proteins tied to neurodegenerative diseases. These proteins have proven particularly challenging for existing predictive tools. Current models operate under a paradigm in which amino acid sequences regulate proteins’ structures, and proteins’ structures dictate their functions. Yet, around 30% of eukaryotic proteins don’t fold into well-defined structures. Since these IDPs and intrinsically disordered regions (IDRs) in other proteins do not conform to the “sequence-structure-function” paradigm, researchers at the University of Copenhagen have begun exploring predictive approaches to help close this gap.
Intrinsically disordered proteins (IDPs) perform a broad range of functions in biology, suggesting that the ability to
design IDPs could help expand the repertoire of proteins with novel functions. Computational design of IDPs with
specific conformational properties has, however, been difficult so far because of their substantial dynamics and struc-
tural complexity.
The researchers headed by Kresten Lindorff-Larsen now describe a general algorithm for designing IDPs with specific structural properties. They have demonstrate the power of the algorithm by generating variants of naturally occurring IDPs that differ in compaction, long-range contacts, and propensity to phase separate. After having experimentally tested and validated their designs, the group analysed the sequence features that determine conformations and captured them in a machine learning model speeding up the algorithm.