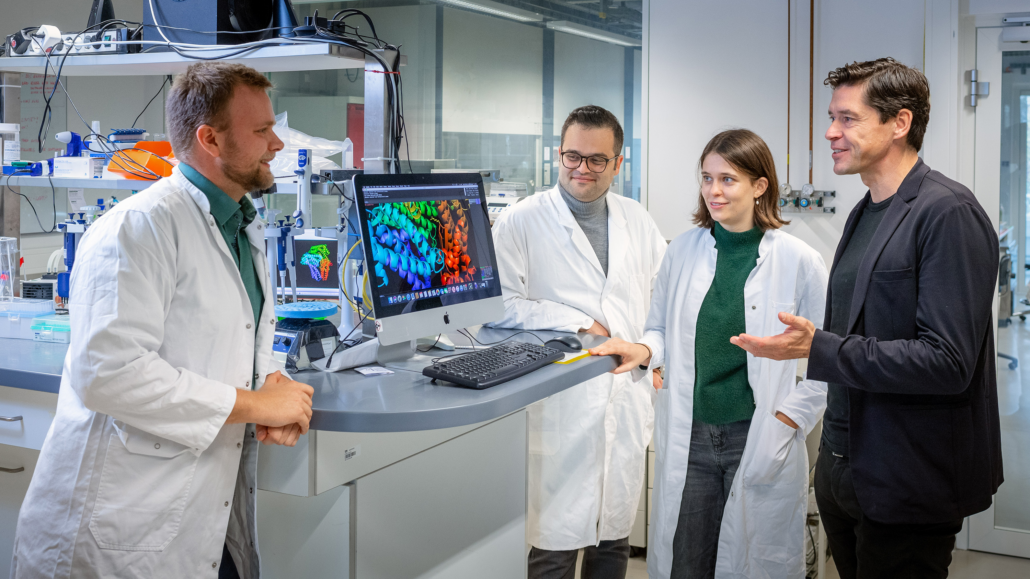
New method set to speed up protein design
Researcher from China, Germany and the US have developed a method for designing large new proteins with pre-defined properties in the lab using Alphafold2.
As an alternative to common generative AI methods, the research group headed by Hendrik Dietz from Technical University of Munich, and Sergey Ovchinnikov at MIT, Boston, used relaxed sequence optimisation for de novo protein design, which enables efficient and robust convergence toward optimal structures on the basis of iterative sequence evolution using gradient descent-based “hallucination” without the need for any form of retraining.
Gradient descent is a common method for model optimisation. In a step-by-step process, it can be used to identify deviations from the desired target function and adjust the parameters until the optimal result is achieved. In protein design, gradient descent can be used to compare the structure of new proteins predicted by AlphaFold2 with the desired protein structure. This allows scientists to further optimise the amino acid chain and the resulting structure that largely determines the stability and function of the protein and depends on subtle energetic interactions.
According to first author Christopher Frank, “We experimentally produced and characterised more than 100 proteins” up to 1,000 amino acids that were validated through high-resolution protein crystallography and cryo–electron microscopy density maps. “Our pipeline can also be used to design synthetic protein-protein interactions, as validated experimentally by a set of protein heterodimers,” rhe researchers write in their paper.
The design process differs from previous approaches. „We have designed the process for new proteins so that we initially ignored the limits of what is physically possible. Usually, only one of the 20 possible building blocks is assumed at each point of the amino acid chain. Instead, we used a variant in which all possibilities are virtually superimposed,“ explained Frank.
This virtual superposition can’t be directly translated into an actually producible protein. But it allows the protein to be iteratively optimised. “We improve the arrangement of the amino acids in several iterations until the new protein is very close to the desired structure,” says Christopher Frank. This optimised structure is then used to determine the amino acid sequence that can actually be assembled to a protein in the lab.
„We were able to show that the structures that we designed are very close to the structures that are actually produced,” stressed Frank. Designing proteins consisting of up to 1,000 amino acids, “brings us closer to the size of antibodies, and – just as with antibodies – we can then integrate several desired functions into such a protein,” concludes team leader Dietz. „These could, for example, be motifs for recognising and suppressing pathogens.”