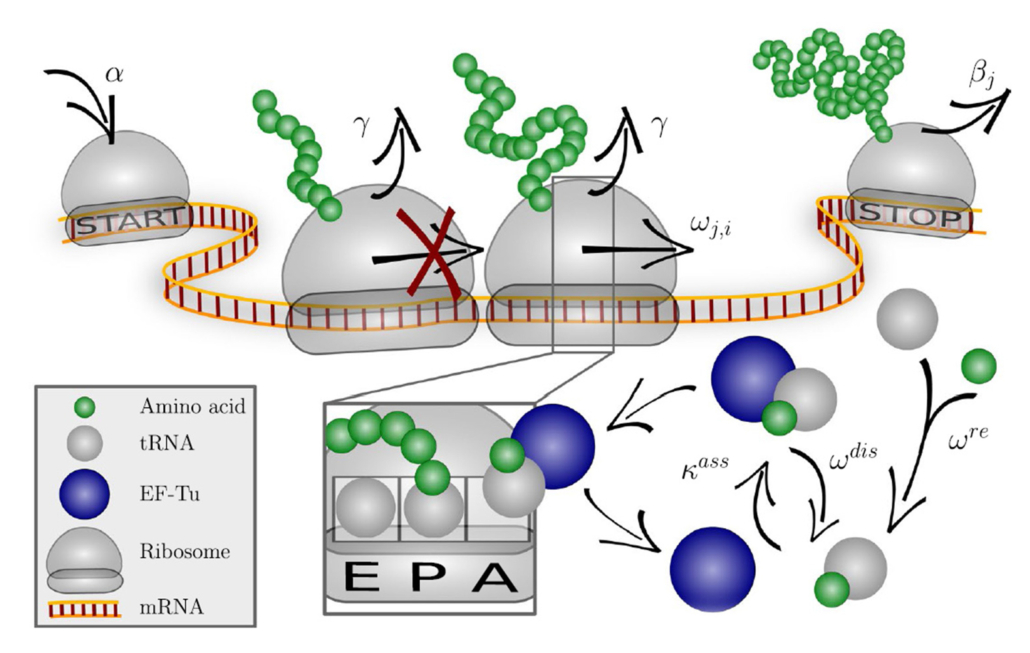
AI improves protein manufacture
Researchers at German regulatory authority Paul-Ehrlich Institute (PEI) have patented a method to accurately forecast biotech-based protein synthesis in host cells.
For unknown reasons, every production cell line or bacterial strain used to express therapeutic proteins recombinantly, has a preference for a certain codon to encode an amino acid. Researchers at PEI and colleagues have created an algorithm that allows for codon optimisation creating higher yields.
Using machine learning and a codon-specific elongation model (COSEM), the team from Adolf-Messer Foundation, Max Planck Institute for Colloids and Interfaces, University of Frankfurt/Main headed by Dr Jan-Hendrik Trösemeier and Dr. Christel Kamp created a protein expression score designed to forecast the protein output and codon optimisation in heterologous protein expression. COSEM simulates ribosome dynamics during mRNA translation and informs about protein synthesis rates per mRNA in an organism- and context-dependent way. Protein synthesis rates from COSEM are integrated with further relevant covariates such as translation accuracy into a protein expression score that the team used for codon optimization
In model organisms, their simulation-based optimisation method was superior to conventional methods. Not only can the protein output increased threefold, but also the accuracy of translation can be improved.
Despite gene optimisation, the algorithm can also be used vice versa: for de-optimisation, i.e. of pathogens. Such attenuation of pathogens is used in vaccine development, to trigger modest immune reactions creating immunity.
Original publication:
Trösemeier JH, Rudorf S, Loessner H, Hofner B, Reuter A, Schulenborg T, Koch I, Bekeredjian-Ding I, Lipowsky R, Kamp C (2019): Optimizing the dynamics of protein expression. Sci Rep May 17 [Epub ahead of print].
https://www.nature.com/articles/s41598-019-43857-5